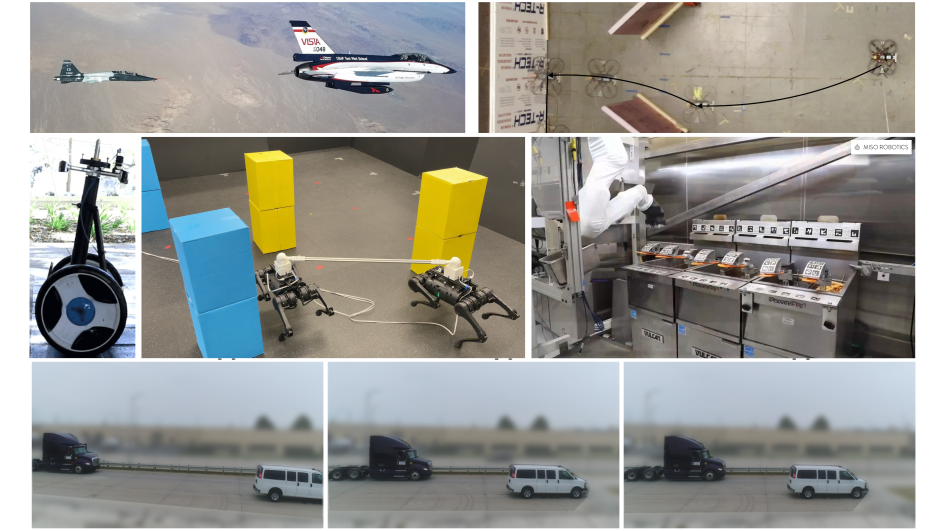
Our research focuses on nonlinear dynamics and control, safety-critical control, and time delay systems with applications to connected automated vehicles, and robotic and autonomous systems.
We established a framework to control autonomous systems – robotic systems and vehicles – with formal safety guarantees based on reduced-order models.
This approach has been implemented on several real-world systems: fixed-wing aircraft, quadrotors, wheeled robots, legged robots, manipulator, and heavy-duty truck.
A summary article is below.
[J32] ![]() |
Cohen et al., Safety-critical control for autonomous systems: Control barrier functions via reduced-order models, ARC 2024 |
We proposed a framework to guarantee safety on robotic systems while satisfying the intention of a human operator, through a learning-based switching law between multiple backup controllers.
We implemented this method on a dual-track robot.
[P32] ![]() |
Janwani et al., A Learning-Based Framework for Safe Human-Robot Collaboration with Multiple Backup Control Barrier Functions, ICRA 2024 |
In collaboration with Dow Chemical, we designed motion plans for quadrupedal robots that allow them to operate in chemical facilities, such as climb up and down in distillation columns.
In collaboration with Miso Robotics, we depeloped a safety-critical control algorithm that allows collision-free cooking with robotic manipulators by minimally modifying existing motion plans.
[J18] ![]() |
Singletary et al., Safety-Critical Manipulation for Collision-Free Food Preparation, RAL/IROS 2022 |
We established a framework for safety-critical control of robotic systems based on reduced-order kinematics.
In case of trivial kinematic equations, this leads to model-free control.
We implemented the method on legged, wheeled and flying robots to execute obstacle avoidance tasks.
[P29] ![]() |
Molnar et al., Safety-Critical Control with Bounded Inputs via Reduced Order Models, ACC 2023 |
[J17] ![]() |
Molnar et al., Model-Free Safety-Critical Control for Robotic Systems, RAL/ICRA 2022 |
We used preference-based learning to tune robust safety-critical controllers for performant and provably safe behavior.
We implemented this method on a quadruped that is controlled by using on-board cameras, with robustness to disturbances and perception errors.
[P26] ![]() |
Cosner et al., Safety-Aware Preference-Based Learning for Safety-Critical Control, L4DC 2022 |
We used self-supervised learning to estimate the uncertainty of perception modules, such as stereo vision, and used this uncertainty estimate for robust safety-critical control.
[P24] ![]() |
Cosner et al., Self-Supervised Online Learning for Safety-Critical Control using Stereo Vision, ICRA 2022 |
We developed a control method to safely transition between various motion primitives (such as lie, stand, walk or jump behaviors) on legged robots.
We implemented this approach on a quadruped.
[P23] ![]() |
Ubellacker et al., Verifying Safe Transitions between Dynamic Motion Primitives on Legged Robots, IROS 2021 |
We established safety-critical controllers that are robust to perception errors, and we implemented them to execute obstacle avoidance tasks by a Segway using on-board cameras.
[P22] ![]() |
Cosner et al., Measurement-Robust Control Barrier Functions: Certainty in Safety with Uncertainty in State, IROS 2021 |
In collaboration with Navistar, we depeloped longitudinal controllers for safe and energy-efficient driving of connected automated trucks.
The controllers leverage road elevation data and information about preceding vehicles from on-board sensors and vehicle-to-vehicle (V2V) connectivity.
We tested the proposed controllers in experiments using a full-scale heavy-duty vehicle, both on a closed test track and on public highways.
We introduced connected cruise control (CCC) strategies by which connected automated vehicles can regulate their logitudinal motions while responding to multiple vehicles ahead of them.
We studied stability and smoothness of driving behavior, the effect of communication, feedback and actuation delays, energy-efficiency and safety.
[P34] ![]() |
Chen et al., Safety-Critical Connected Cruise Control: Leveraging Connectivity for Safe and Efficient Longitudinal Control of Automated Vehicles, ITSC 2024 |
[P30] ![]() |
Molnar et al., On the Safety of Connected Cruise Control: Analysis and Synthesis with Control Barrier Functions, CDC 2023 |
[J30] ![]() |
Shen et al., Energy-efficient Reactive and Predictive Connected Cruise Control, IEEE IV 2024 |
[P27] ![]() |
Molnar et al., Input-to-State Safety with Input Delay in Longitudinal Vehicle Control, TDS 2022 |
[J08] ![]() |
Molnár et al., Application of predictor feedback to compensate time delays in connected cruise control, IEEE ITS 2018 |
We studied the behavior of mixed traffic systems where human drivers and connected automated vehicles (CAVs) coexist.
By leveraging connectivity, CAVs can regulate their motions such that they make the overall traffic flow smoother, that ultimately mitigates traffic jams and improves mobility.
In collaboration with Ford, we depeloped an on-board traffic prediction method that provides speed previews for vehicles via vehicle-to-vehicle (V2V) connectivity.
Prediction was implemented on a physical vehicle and tested in real highway traffic.
We developed a control framework to achieve formal guarantees of safe behavior for control systems that have time delays in their control loops.
The frameworks covers systems with input delays and state delays.
We established controllers for epidemiological models that describe the spread of Covid-19.
These controllers give guidance on the required human intervention for mitigating the spread of the virus and for providing safety with respect to the level of infection or hospitalization.
Click on the image for a press release of our work.
We studied the behavior of delayed dynamical models describing machine tool vibrations in metal cutting processes such as turning and milling.
Through stability and bifurcation analysis, we identified those choices of technological parameters that enable manufacturing engineers to avoid harmful vibrations (chatter) during the cutting process.
We investigated predictor feeback control techniques to overcome the detrimental effects of time delays in control loops (i.e., sensory, communication, feedback and actuation delays).
We leveraged these methods in the context of controlling connected automated vehicles, epidemiological models and safety-critical time delay systems.